In the first part of my look at Nvidia (NVDA), I stated that most of the hype surrounding the company comes from the belief it will be the leader in the parallel computing era.
Jefferies analyst Mark Lipacis refers to this as “the fourth tectonic shift in computing.” The first computing era was that of mainframes. This was followed by a move to minicomputers, then PCs, and finally mobile phones.
Now, according to Lipacis, we are moving into the era of parallel computing. And that’s going to pay off big for Nvidia…
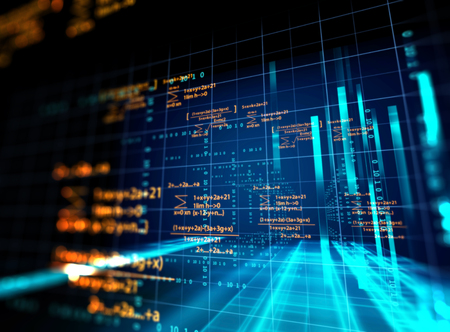
Graphics processing units, or GPUs, allow faster computing because they use matrix calculations rather than linear calculations. This is known as parallel computing. To process this much information, the GPUs need hundreds of “cores” as opposed to the handful that sit on central processing units (CPUs).
In simple terms, the more GPUs that can be strung together, the more computing power there is. The largest computers currently will have around 10,000 GPUs.
Nvidia’s Plan Comes Together
Nvidia did not end up on top of the AI world at the moment by chance. The company has literally been working for decades to command the parallel computing ecosystem, much as Apple (AAPL) ended up controlling the mobile phone era.
In the popular 80s TV show, The A-Team, the leader of the eponymous squad was Colonel John “Hannibal” Smith, played by actor George Peppard. His catchphrase became very popular: “I love it when a plan comes together.”
I feel certain Nvidia’s co-founder and CEO Jensen Huang says that phrase every day.
Looking back, it is easy to see how Nvidia’s plan came together.
In 2006, the company invented an interface called CUDA, which is needed to write programs for its GPUs. Most AI engineers now use CUDA, which means it is difficult for them to work with other parallel computing chips and they are pretty much locked into the Nvidia ecosystem.
The next key step in Nvidia’s plan came with the $6.9 billion acquisition of Mellanox in 2019. This is a company that sells its specialized Infiniband cables that connect GPUs to each other. A single GPU is fine for a gaming computer, but to train a large language model with billions of parameters you need to string together thousands of GPUs. For example, the Microsoft supercomputer used to train ChatGPT has more than 10,000 Nvidia GPUs strung together via Mellanox cables.
Apple has dominated the mobile phone era by selling both the hardware and software, which is just what Nvidia’s plan wants to accomplish in parallel computing.
Next let’s look at what is powering Nvidia’s rise to the top of the AI world, its H100 chip. It is based on a new Nvidia chip architecture called “Hopper,” named after the American programming pioneer Grace Hopper.
Hopper was the first architecture optimized for “transformers,” the approach to AI that underpins OpenAI’s “generative pre-trained transformer” chatbot. It was Nvidia’s close work with AI researchers that allowed it to spot the emergence of the transformer in 2017 and start tuning its software accordingly.
The unusually large chip is an “accelerator,” designed to work in data centers. It has an incredible 80 billion transistors—five times as many as the processors that power the latest iPhones! While this new chip is twice as expensive as its predecessor, the A100 (released in 2020), early adopters say the H100 boasts at least three times better performance.
And while the timing of the H100’s launch was a bit lucky, Nvidia’s breakthrough in AI can be traced back directly to its innovation in software—the aforementioned Cuda software, created in 2006, allows GPUs to be repurposed as accelerators for other kinds of workloads beyond graphics.
Again, I want to emphasize how Nvidia was prepared for this AI-led generation of computing. Even back in 2012, Ian Buck, currently head of Nvidia’s hyperscale and high-performance computing business, said, “AI found us.”
Nvidia today has more software engineers than hardware engineers. This enables it to support the many different kinds of AI frameworks that have emerged and make its chips more efficient at the statistical computation needed to train AI models.
GPUs’ Skyward Growth Path
Jefferies analyst Mark Lipacis said: “The data shows that each new computing model is 10 times the size of the previous one in units. If cell phone units are measured in the billions, then the next one must be in the tens of billions.”
Lipacis’s analysis suggests much higher revenues are on the way for Nvidia—and he looks to be correct. Here’s why…
Language models get better by increasing in size. GPT-4, the latest system powering ChatGPT, was trained on tens of thousands of GPUs, and the forthcoming GPT-5 is reportedly being trained on 25,000 Nvidia GPUs.
The tech research firm TrendForce estimates that the GPU market will grow at a compound annual growth rate of 10.8% between 2022 and 2026, as companies scale up capacity to meet demand.
Meanwhile, a lack of hardware (GPUs) could put a limit on the number of models that can be trained. This shortage of supply enables Nvidia to charge a high price for its GPUs. Analysts are expecting its operating margin to rise to 41% next year, compared with a five-year average of 29%.
It was not long ago—in 2022—when Nvidia released the H100, one of the most powerful processors it had ever built…and one of its most expensive, costing about $40,000 each.
The launch seemed badly timed, just as technology businesses sought to cut spending amid recession fears. But then in November 2022, ChatGPT launched, and the tech world changed in an instant. OpenAI’s hit chatbot created instant demand. ChatGPT’s popularity triggered a rush among the world’s leading tech companies and start-ups to obtain the H100, which CEO Huang describes as “the world’s first computer [chip] designed for generative AI”.
Nvidia had the right product—the H100—at the right time. The company had just begun manufacturing it at scale a mere few weeks before ChatGPT debuted.
In summary, Nvidia arguably saw the future before everyone else with its pivot into making GPUs programmable. It spotted the opportunity and bet big, allowing it to easily outpace its competitors.
That’s why today Nvidia sits on top of the AI mountain, with probably a two-year lead over its rivals. Whether it can continue to see the future and remain ahead of the competition is the only open question. But I would not bet against them.
Nvidia’s stock is a buy on any weakness, ideally below $400 a share.